Researchers built an AI model to predict stocks’ earnings better than Wall Street. Here it is.
Feed ChatGPT some financial statements, do some chain-of-thought prompting, and it can give you better forecasts than an investment bank full of analysts.
20th December 2024 09:32
Reda Farran from Finimize
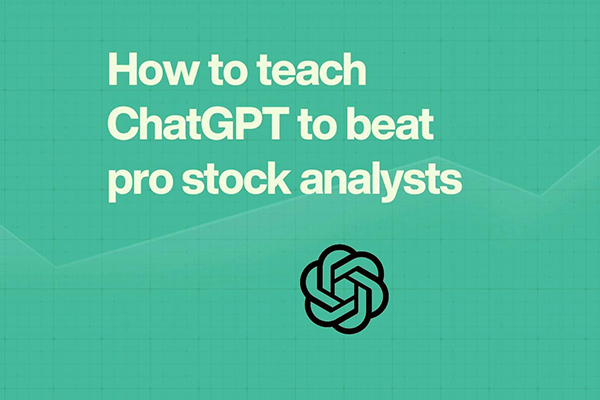
Back in May, three University of Chicago scholars published a fascinating research paper that tested whether ChatGPT can perform financial statement analysis as effectively as the pros. They found that with some fairly light prompting, the AI sized up those complicated reports and turned them into earnings forecasts that were more accurate than Wall Street’s. These predictions were then used to create model investment portfolios that, when backtested, delivered huge excess returns. The best part: the researchers made their model publicly available. So, let me break down how it works – and how you can apply it to your own portfolio.
Thesis
- The researchers fed ChatGPT thousands of financial statements, stripped of dates and company names, from a database of more than 15,000 companies from 1968 to 2021. Each statement was a single input – meaning, no historical context or longer-term firm data was made available to the model.
- They then used "chain-of-thought prompting" to ask ChatGPT a series of questions and get it to predict whether each company’s earnings would be up or down in the following year and whether the magnitude of the change would be small, medium, or large. They also asked the AI how sure it was of its prediction.
- The model’s forecasts were accurate 60.4% of the time, compared to the 52.7% accuracy of professional human analysts’ estimates made one month after financial statements were released. Even more impressively, ChatGPT beat the forecasts analysts made six months after the release, even though those estimates benefited from more timely information.
- The researchers built long-short model portfolios based on the companies for which ChatGPT was highly confident would see big earnings changes. In backtests, those portfolios performed much better than the broader stock market and delivered impressive risk-adjusted returns.
Risks
- ChatGPT can lead you astray. That’s why you shouldn’t use it in isolation when making investment decisions. It’s a useful tool that can supplement, not replace, your own thorough research. Remember: the AI’s predictions were wrong 40% of the time.
- ChatGPT’s accuracy has decreased, on average, by 0.1 percentage points per year, which suggests that it has become increasingly difficult to predict future earnings using only numerical information.
- Earnings forecasts become less relevant throughout the year as companies’ quarterly results get released and as management teams provide short-term profit guidance.
- For certain early-stage companies, what happens to earnings next year is a lot less relevant than the bigger, longer-term outlook of the firm and the wider industry.
PART I: HOW TO TURN CHATGPT INTO A FINANCIAL ANALYST
The researchers instructed ChatGPT to act as a stock analyst, evaluating financial statements by identifying significant changes in key items, calculating ratios, and writing economic narratives to contextualize the assessment. The model was then tasked with predicting whether companies' earnings would increase or decrease in the next year, estimating the magnitude of the change, and gauging its own confidence in the forecast.
The researchers – Alex Kim, Maximilian Muhn, and Valeri Nikolaev – fed a database of balance sheets and income statements, covering over 15,000 companies across 54 years, into what was then the latest version of ChatGPT (GPT-4-0125-preview). They were careful to control what the AI did and didn’t know about the companies, so they could get an accurate sense of its analytical abilities.
For example, company cash flow statements weren’t directly included in the AI inputs, but they can be extrapolated from the other two statements. What’s more, the data was stripped of dates and corporate names to make sure ChatGPT couldn’t use its knowledge of major market events (for example, the global financial crisis) or company news to adjust its predictions.
The researchers also excluded text summaries that often accompany these statements – for example, management discussion and analysis. That’s because their primary goal was to assess ChatGPT's ability to analyze and synthesize purely numerical data.
Consistent with US reporting requirements, the researchers poured two years of balance sheet and three years of income statement data into the AI, but each as an individual input. In other words, no historical context or longer-term company data was made known to the model. Next, they used a technique called "chain-of-thought prompting" to ask ChatGPT a series of questions. This approach aims to mimic human-like reasoning by asking the AI to break down its thought process, enhancing the accuracy and coherence of its responses.
Step-by-step, the researchers:
- Told ChatGPT to take on the role of an analyst whose task is to perform financial statement analysis.
- Asked the model to identify notable changes in certain financial statement items and calculate key financial ratios, without explicitly limiting the set of ratios that need to be computed. They told the AI to first state the formulas before performing the calculations.
- Instructed ChatGPT to write economic narratives that explained the outputs of the financial analysis (this turned out to be crucial, as you’ll see later).
- Directed the model to use its analysis to predict whether each company’s earnings would increase or decrease in the subsequent year and whether the magnitude of the change is expected to be small, medium, or large. The model was also asked to assess its confidence in these predictions, producing a score that ranges from zero (random guess) to one (perfectly informed).
- Asked the model to write a paragraph that explains the rationale behind each prediction.
Overall, these instructions are designed to mimic how human analysts process financial information and come up with predictions.
PART II: THE RESULTS OF THE AI’S ANALYSIS – AND HOW IT STACKS UP AGAINST THE HUMANS
The model’s forecasts were accurate 60.4% of the time, compared to the 52.7% accuracy of the estimates that professional human analysts made one month after financial statements were released. Even more impressively, ChatGPT beat the forecasts analysts made six months after the release, even though those estimates benefited from more timely information.
Before we look at how ChatGPT performed, it’s worth first assessing how the benchmark – human analysts – fared. The researchers examined analyst forecasts drawn from the same database they used to pull the financial statements. The analyst sample includes data from 1983 to 2021, encompassing nearly 40,000 observations from more than 3,000 companies.
To ensure the analyst forecasts properly reflect the most recent company results, the researchers used consensus estimates taken one month after the release of financial statements. They also used three-month and six-month ahead consensus forecasts as alternative benchmarks. Here’s what they found:
- One-month ahead analyst forecasts achieved an accuracy of 52.7% in predicting the direction of future earnings, which is better than the 49.1% accuracy of a naïve model that simply extrapolates the prior year’s profit change.
- These results highlight the fact that changes in earnings are very hard to predict, even for sophisticated financial analysts. After all, a 52.71% accuracy rate is only slightly better than a coin toss.
- Three- and six-month ahead forecasts achieved a meaningfully higher accuracy of 56% and 56.7%, respectively, which makes sense, since they incorporate more timely information.
When it came to ChatGPT’s predictions, the model’s forecasts were 52.3% accurate when using simple, non-chain-of-thought prompts. That’s on par with one-month ahead estimates by human analysts. However, once the researchers used the chain-of-thought prompting method described above, the model’s accuracy increased to a whopping 60.4% – much better than the human analysts. What’s even more remarkable is that ChatGPT was more accurate than analyst forecasts made six months after the release of financial statements. That is, even with one or two additional quarters of actual results and management guidance for upcoming earnings, analysts still underperformed ChatGPT.
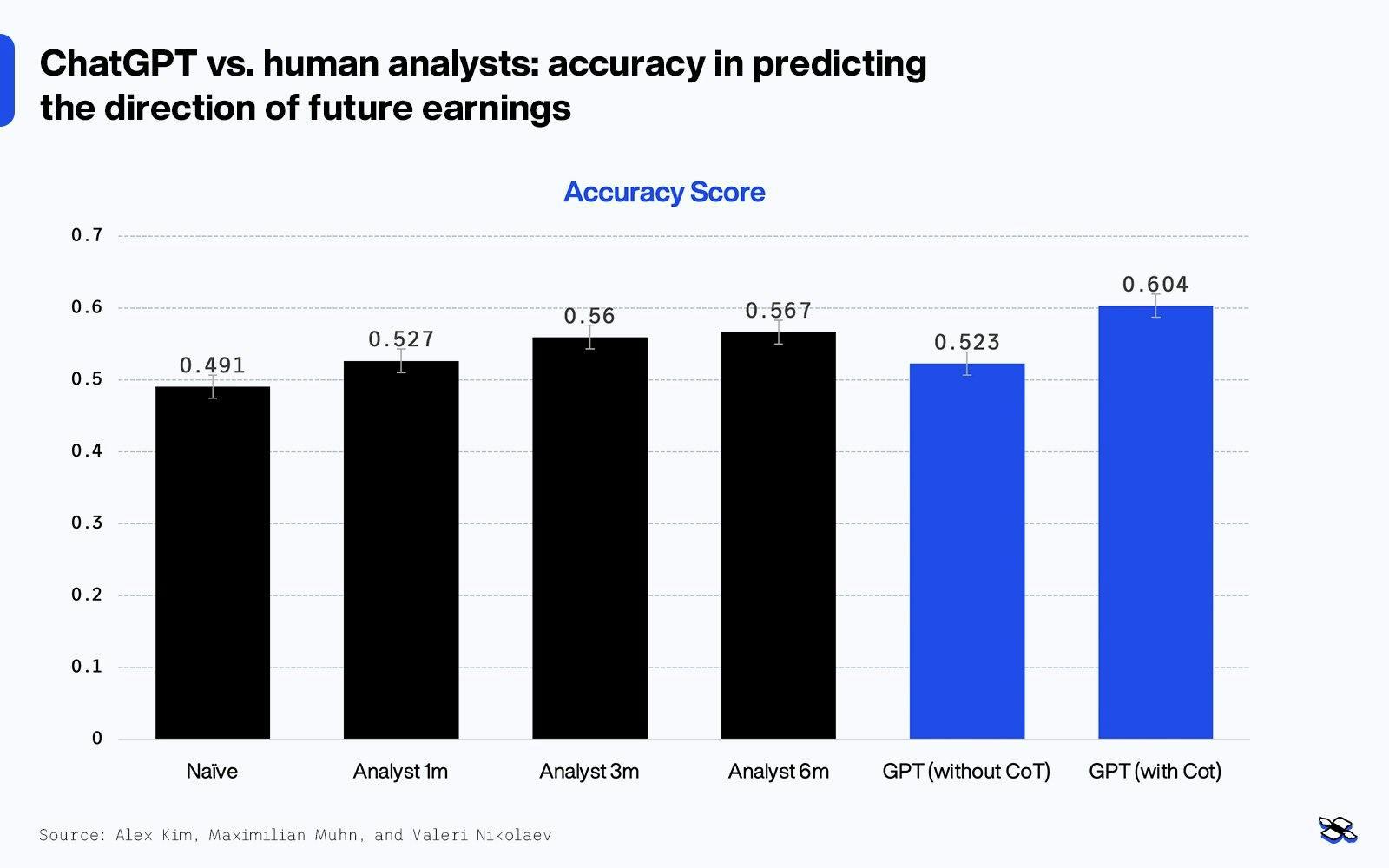
ChatGPT versus human analysts: accuracy in predicting the direction of future earnings. Source: Alex Kim, Maximilian Muhn, and Valeri Nikolaev.
Now, remember that the researchers also asked ChatGPT to assess its confidence in its predictions. As expected, forecasts made with higher confidence were more accurate than those with lower confidence. Also recall that ChatGPT was tasked with predicting whether the magnitude of earnings changes would be small, medium, or large. The forecasts were 62% accurate when the model predicted big changes and dropped to 60.2% for little changes. In summary: ChatGPT’s forecasts were more accurate when it expressed higher confidence in its predictions and/or anticipated bigger earnings changes.
Finally, the researchers also compared ChatGPT’s performance to a machine-learning model designed specifically for predicting earnings. While I won’t delve into the technical details, the key takeaway is that ChatGPT’s accuracy was comparable to – and in some cases, slightly better than – that of the specialized machine-learning model.
PART III: A FEW GENERAL TAKEAWAYS FROM THIS RESEARCH
ChatGPT and other AI models like Alphabet's Gemini can be used in lots of ways in the finance world, from analyzing annual reports to forecasting earnings and predicting stock price movements based on news events. This stems from their broad knowledge and ability to process huge amounts of data efficiently, which can help less-experienced investors make better-informed decisions. The model’s accuracy improves with chain-of-thought prompting, highlighting the importance of guiding AI reasoning step by step.
1) ChatGPT can be used in lots of ways in the finance world.
Processing financial data and forecasting earnings is challenging, even for the most skilled analysts. The researchers’ findings show that general-purpose large language models like ChatGPT can be an asset in performing these tasks. These AI systems stand out for their broad knowledge across many fields and their ability to quickly and efficiently process huge amounts of data. They have the potential to democratize financial statement analysis in a way that can empower less-experienced investors to make better-informed decisions.
Their capabilities go beyond earnings forecasts too – these AI models have lots of other finance applications. For example, one research paper showed that ChatGPT can accurately predict stock price movements using news headlines. In another study, two researchers from the Federal Reserve (Fed) found that ChatGPT came closest to humans in figuring out if the central bank’s statements were “dovish” (and leaning toward lower interest rates) or “hawkish” (and leaning against). Remarkably, the AI was also able to use its analysis to predict future macroeconomic shocks linked to shifts in the Fed’s tone. And in a popular Finimize piece I wrote not too long ago, I showed how investors can use ChatGPT to analyze a stock.
These are just examples of some of the research that demonstrates how AI can be effectively used in investing. There are many others too – with undoubtedly loads more to come.
2) Chain-of-thought prompting is really powerful.
Ever since ChatGPT’s ground-shaking release, there’s been a flurry of prophecies about AI and its potential to replace humans in “knowledge work”. And I don’t think anyone would argue against the notion that AI is going to replace some jobs (it’s already happening), but there’s certainly debate about how many and how fast. I personally believe it’s more important than ever to learn how to effectively use AI to become more efficient in whatever job you hold. I’m willing to bet that pros who have a deep understanding of their field, soft skills, and the ability to effectively leverage AI will be more secure in their careers.
And to that end, it’s worth noting how useful ChatGPT became over the course of the study, with some chain-of-thought prompting. Recall that the AI's accuracy in predicting the direction of earnings improved by nearly eight percentage points when this method was used, over simpler prompts. So next time you use ChatGPT to solve a problem, experiment with chain-of-thought prompts that guide the AI to break down its reasoning step by step. You may find a big improvement in the accuracy, clarity, and reliability of its responses.
3) ChatGPT isn’t the only smart bot on the block.
Sure, ChatGPT is probably the first name that springs to mind when you think about AI chatbots, but there are plenty of others out there – Anthropic’s Claude and Alphabet Inc Class A (NASDAQ:GOOGL)’s Gemini (previously called Bard), to name just two.
To test the capabilities of Alphabet’s bot, the researchers ran the same earnings prediction experiment with Gemini Pro 1.5 and ChatGPT competing side-by-side, using a random 20% sample of the full dataset. And it was a close contest: Gemini’s forecasts were correct 59.2% of the time, just shy of ChatGPT’s 61.1%. Their outputs overlapped quite a bit, with only about 6% of their earnings forecasts pointing in opposite directions.
The takeaway is that Gemini performed nearly as well as ChatGPT and, perhaps more importantly, managed to beat human analysts’ forecasts. This shows that Alphabet’s AI chatbot shouldn’t be dismissed just because of ChatGPT’s ubiquity.
In fact, a little over a year ago, I pitted ChatGPT against Gemini (called Bard at the time) to see which was more useful for analyzing stocks. While ChatGPT came out ahead based on my simple scoring system, I highlighted several key advantages that Bard offered. Ultimately, I believe the focus shouldn’t be on striving to select the perfect chatbot but on embracing the use of AI in the first place. While the booming tech tools should never be used in isolation, they can be exceptionally handy in making your investing life easier.
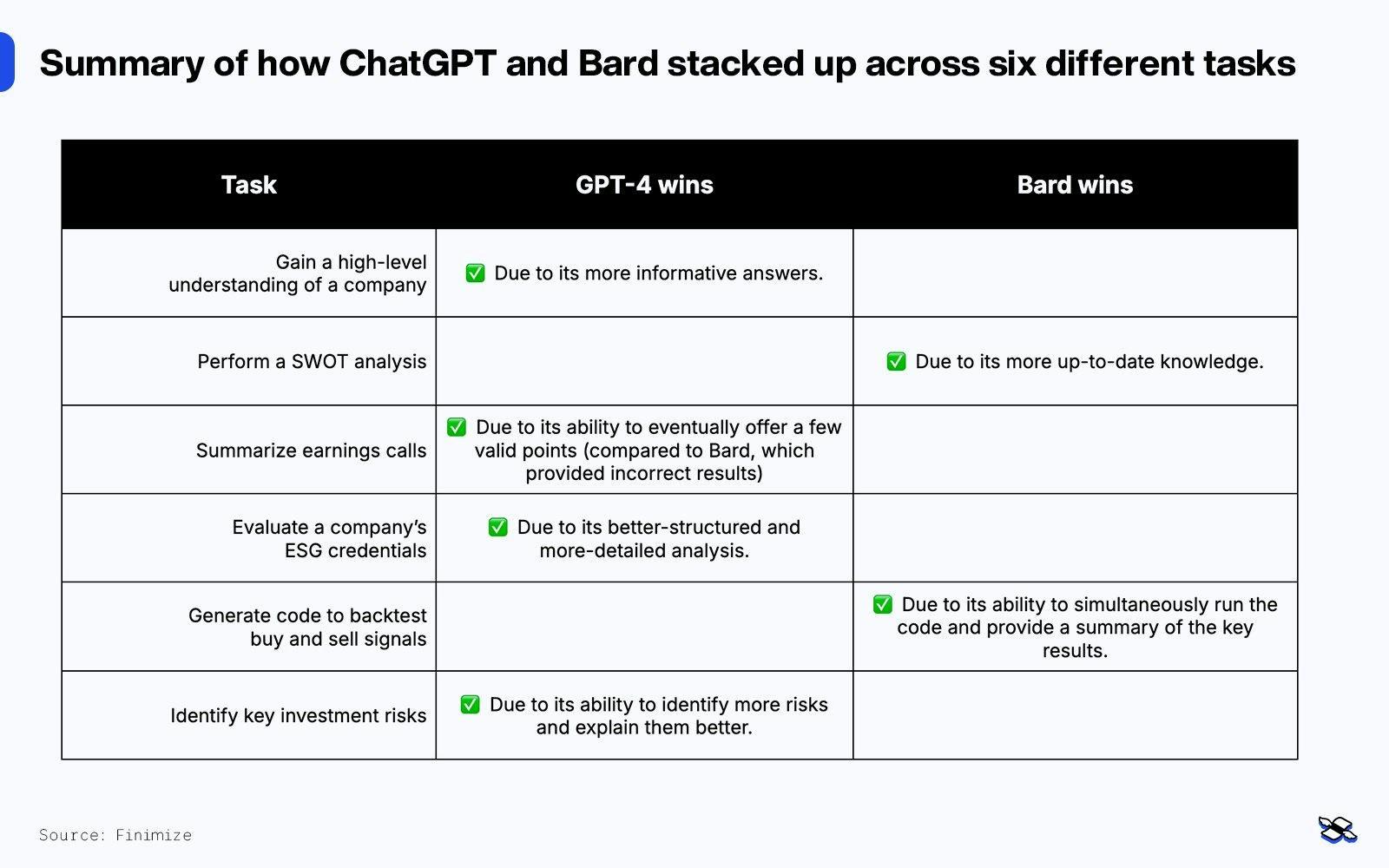
Summary of how ChatGPT and Bard stacked up across six different tasks. Source: Finimize.
PART IV: SOME TRULY USEFUL TAKEAWAYS FOR INVESTORS
Here's where things get really interesting. The researchers built long-short model portfolios based on the forecasts that ChatGPT made with the highest confidence – essentially, betting on the gains or losses on each of those stocks. And in backtests, these portfolios significantly outperformed the broader stock market.
This is how they did it:
- Portfolios were constructed on June 30 of each year, allowing plenty of time for the market to digest companies' annual reports (which are typically released by the end of March). Each portfolio was held for one year – until the following June 30.
- For each fiscal year, the researchers filtered for stocks that ChatGPT predicted would see an increase in earnings, with the expected change categorized as either “medium” or “large”.
- The selected stocks were then ranked based on ChatGPT’s confidence in its predictions (recall that the researchers had asked the model to assess its confidence in its forecasts using a score ranging from zero to one).
- The model portfolio went long (bought) the top-ranked stocks, ensuring that the total number of firms included in the portfolio made up 10% of the sample for that year. For example: if, in a given year, the researchers fed 3,000 companies’ financial statements into ChatGPT, the final long portfolio would contain 10% x 3,000 = 300 stocks.
- The exact same process was repeated for the short portion of the portfolio, but using stocks that ChatGPT predicted would see a decrease in earnings.
- Two different methods were used to assign weights to the stocks in the portfolios: market-cap weighting and equal weighting.
Essentially, the model portfolio buys stocks that ChatGPT is highly confident will experience a modest or big increase in earnings, while shorting stocks it’s confident will experience a moderate or large decrease in earnings. The research paper doesn’t specify the average number of stocks included in these portfolios. However, based on other details provided (e.g. the time span of the data and the sheer number of observations), I estimate that each long and short portfolio contained 200 to 300 stocks.
Now, onto the results. As expected, the long portfolio outperformed the short one by a big margin, leading to strong returns for the combined long-short portfolio. Specifically, it delivered an annualized return of 15.4% on an equal-weighted basis and 6.7% on a market-cap-weighted basis. The former’s superior performance suggests that ChatGPT adds more value with its predictions of small stocks’ earnings. What’s more, the long-short portfolio was quite resilient, holding its own even during market downturns, as shown below.
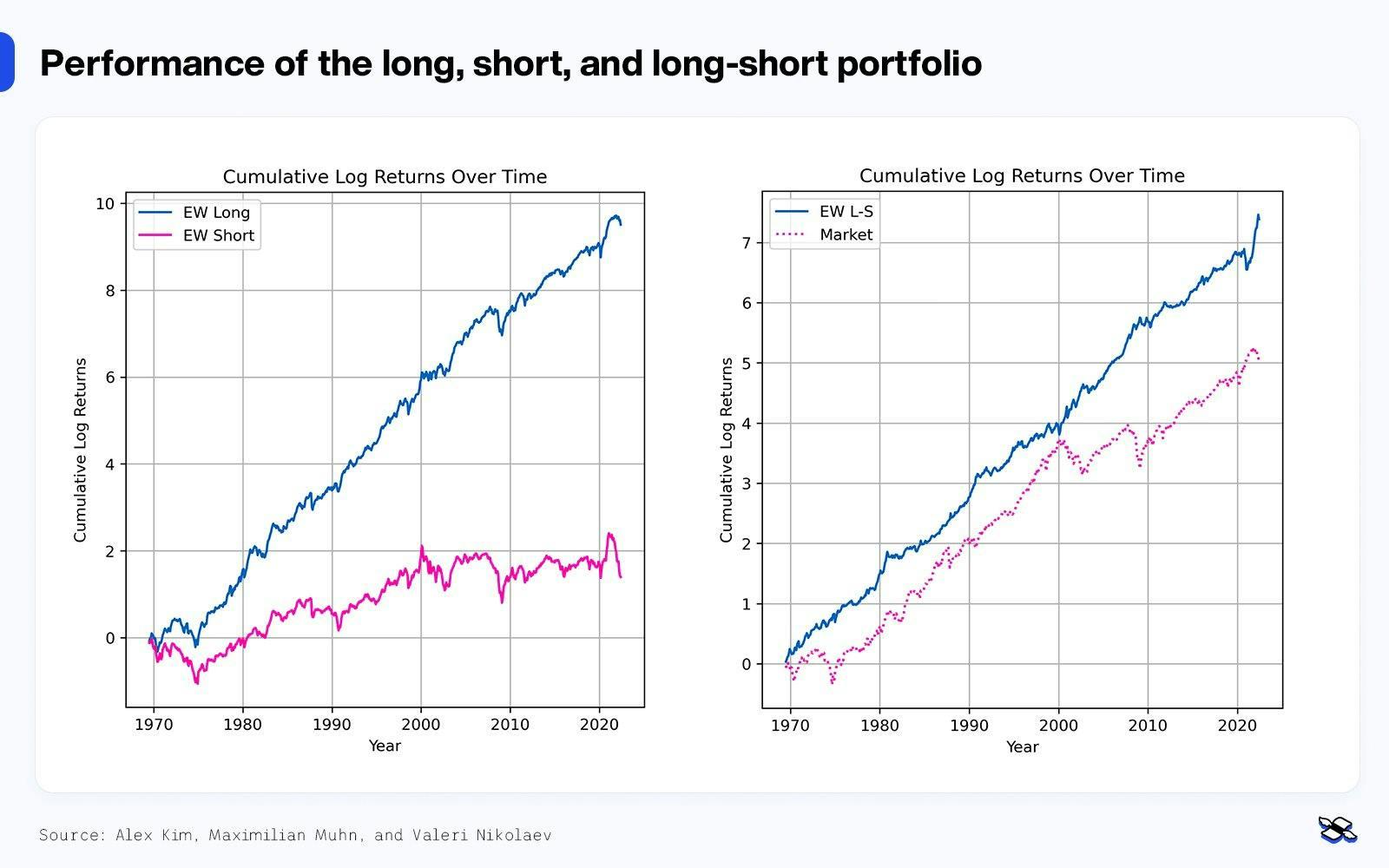
The performance of the long, short, and long-short portfolios (all constructed by equally weighting the stocks). Source: Alex Kim, Maximilian Muhn, and Valeri Nikolaev.
To assess the portfolio’s risk-adjusted returns, we can look at its Sharpe ratio. This is calculated by dividing the portfolio’s annualized excess returns (that is, returns above that of a so-called risk-free asset like US Treasury bills) by its annualized volatility. It basically tells you how the portfolio performed per unit of risk. And here’s what’s impressive: the researchers’ long-short model portfolio achieved a Sharpe ratio of 3.4 on an equal-weighted basis and 1.5 on a cap-weighted basis. For context, investors generally consider a Sharpe ratio between 1 and 2 as good, between 2 and 3 as very good, and above 3 as exceptional.
Finally, to assess how the long-short portfolio fared relative to the market, we can look at its “alpha”. Without delving deep into the technical details, alpha represents the portfolio's excess return above the market and the risk-free rate – beyond what can be explained by its exposure to market risk (as measured by “beta”). The researchers’ long-short model portfolio achieved an annualized alpha of 15.8% on an equal-weighted basis and 8.9% on a cap-weighted basis. And that’s very impressive.
PART V: FINALLY, HOW TO APPLY THESE FINDINGS TO YOUR OWN INVESTING
The researchers developed an interactive GPT (essentially a customized version of ChatGPT) that can perform financial statement analysis and predict a company’s earnings direction using methods similar to those outlined in their study. The insights that it provides can help make your investing life a lot easier, especially if you want to get a quick, decent overview of a company you’re considering adding to your portfolio.
You can access the researchers’ model here, but you’ll need a paid ChatGPT Plus subscription to do so. When you upload an annual financial report to the GPT, it conducts a series of insightful analyses to help you better understand the company. This includes:
- Summarizing the firm’s business model, revenue sources, and risk factors
- Explaining what happened to major financial statement items (year-over-year) and why
- Calculating key financial ratios (profitability, liquidity, efficiency, etc)
- Predicting whether the company’s earnings will increase or decrease in the subsequent year and whether the magnitude of the change will be small, medium, or large
- Assessing its own confidence in its prediction
- Explaining its rationale for the forecast
These are useful insights that can help make your investing life a lot easier, especially if you want to get a quick, decent overview of a company you’re considering putting your money in. The GPT will even invite you to ask follow-up questions.
What’s more, the researchers’ model can enhance other ways to use ChatGPT to analyze a stock (something I wrote about here). But bear in mind that an AI tool should never dictate where you put your money – at least not in isolation. In other words, a chatbot’s analysis can be useful, but you should always conduct your own research. Remember, the GPT’s predictions were right about 60% of the time – but that means it was wrong 40% of the time.
Now, maybe you’re wondering about potentially replicating the researchers’ model portfolios – which, if you recall, delivered highly impressive investment returns. And, yeah, in theory, that’s possible since you have access to the GPT. But in practice, feeding the bot thousands of annual reports, organizing its predictions, and implementing a long-short portfolio with hundreds of stocks is an incredibly time-consuming and impractical task. Perhaps this could be automated with coding, but, frankly, that’s above my pay grade.
Having said that, the GPT’s predictions could be used to form specific portfolios in other ways. Imagine, for example, you’re interested in investing in a particular theme. You could, say, find a suitable ETF focused on that theme and use the GPT to form a long-short portfolio based on the fund’s holdings. Here’s how:
- Input the latest annual reports for each of the ETF’s holdings into the model, processing one company at a time and recording the GPT’s predictions.
- Filter for stocks where the model predicts a “large” increase in earnings (that’s a narrower approach than the researchers took: they included medium and large increases).
- Rank the filtered stocks by the GPT’s confidence in its predictions.
- Buy the top, say, 10 stocks with the highest confidence scores.
- Short the 10 stocks with the highest confidence scores where the GPT predicts a “large” decrease in earnings.
- Repeat every year on 30 June.
Essentially, you’re creating a long-short portfolio that’s centered on a specific theme, using the predicted earnings of the underlying companies to select the stocks. Since a long-short portfolio typically has low net market exposure, it should, in theory, exhibit lower volatility. This approach could deliver strong risk-adjusted returns, provided the long positions outperform the short ones. If you want to create a long-only portfolio, simply follow the steps outlined above, but skip the fifth step.
Full disclosure: I haven’t tried or backtested this approach, so I can’t say whether it will work in practice. What I really wanted to emphasize is how the GPT can help you narrow down a long list of stocks into a long-short portfolio using a method supported by academic research. If this sounds too complex or abstract, that’s perfectly fine – you can simply use the GPT to help you analyze individual stocks, as described earlier.
Reda Farran is an analyst at finimize.
ii and finimize are both part of abrdn.
finimize is a newsletter, app and community providing investing insights for individual investors.
abrdn is a global investment company that helps customers plan, save and invest for their future.
These articles are provided for information purposes only. Occasionally, an opinion about whether to buy or sell a specific investment may be provided by third parties. The content is not intended to be a personal recommendation to buy or sell any financial instrument or product, or to adopt any investment strategy as it is not provided based on an assessment of your investing knowledge and experience, your financial situation or your investment objectives. The value of your investments, and the income derived from them, may go down as well as up. You may not get back all the money that you invest. The investments referred to in this article may not be suitable for all investors, and if in doubt, an investor should seek advice from a qualified investment adviser.
Full performance can be found on the company or index summary page on the interactive investor website. Simply click on the company's or index name highlighted in the article.
Editor's Picks